Why it matters: As first-person (FPV) drone racing grows in popularity, AI implementations have continued improving their results against human pilots. While a great deal of uncharted territory remains for this area of research, it could eventually impact various real-world applications for autonomous drones.
In 2021, researchers from the University of Zurich debuted an autonomous drone control system that could outfly human pilots on race tracks. In the two years since then, they've developed a successor they claim defeated three world-champion FPV drone racers.
The emerging sport tasks competitors with flying a small drone through a series of gates in the correct order as quickly as possible, with the video feed from the drone's camera connected to the pilot's goggles. The quick reflexes and high degree of skill accomplished racers exhibit push the boundaries of drone maneuverability, making them an interesting target for research into autonomous control systems.
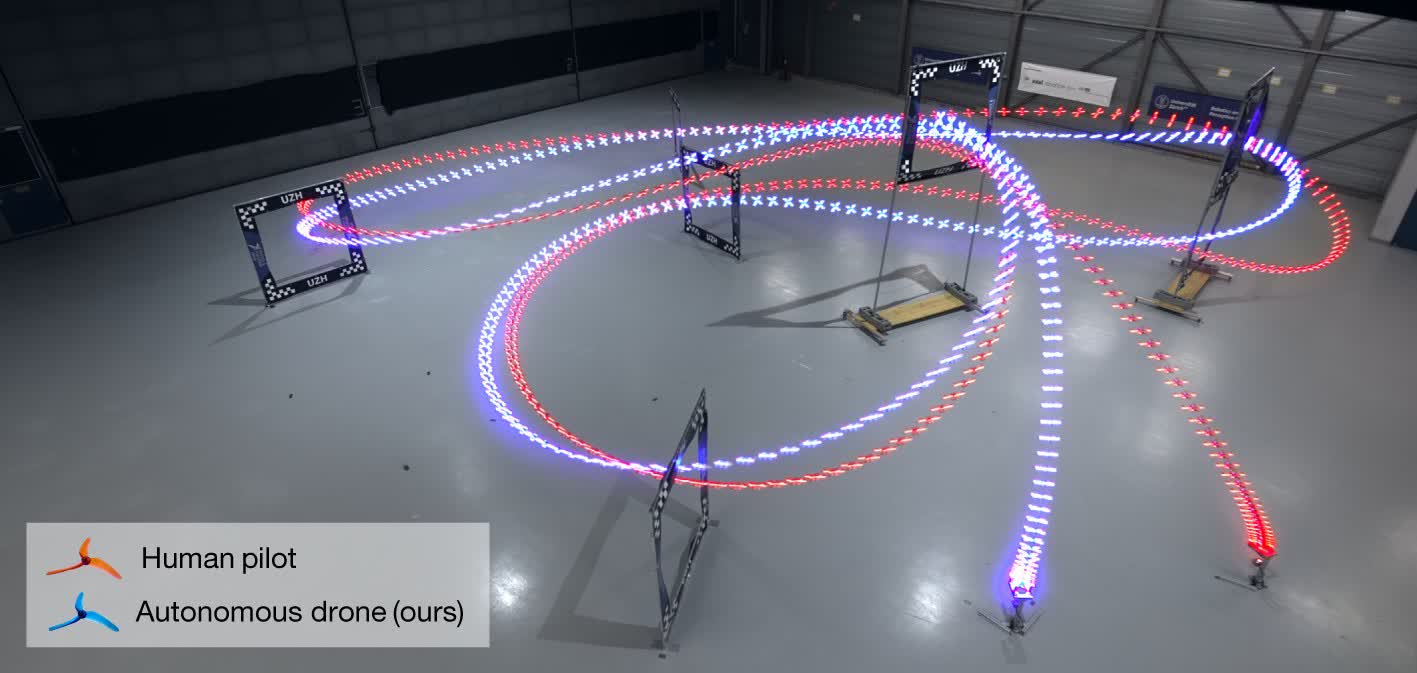
Training the AI, called Swift, involved a neural network and data received from an onboard computer, a camera, and an inertial sensor. Swift posted record track times during the test, defeating three international world champions, primarily because it took far tighter turns than the human pilots. Research into autonomous racing systems is almost as old as drone racing, but the University of Zurich's recent results have reached a new level.
Possibly the most striking factor is that, while the human racers spent a week training on the test course, the AI training process only took around an hour on a standard workstation desktop. Two possible advantages in the drone's favor are that it processes information faster than the racers' brains and senses inertia in a way that humans don't. However, Swift's video feed was only 30Hz while the pilots' cameras refreshed at 120Hz, offering them more visual data.
A significant caveat is that Swift has only been tested on one indoor course, whereas drone races are held in various indoor and outdoor settings. It's unclear how autonomous systems like Swift would handle factors like wind or changes in lighting conditions, so there's certainly room for future research.
The results of this and other experiments could have implications reaching far beyond drone racing. They might help improve how self-flying drones navigate real-world environments for purposes like delivery, search and rescue, warfare, and more.
https://www.techspot.com/news/100007-ai-beats-world-champion-first-person-drone-racers.html